News and Announcements
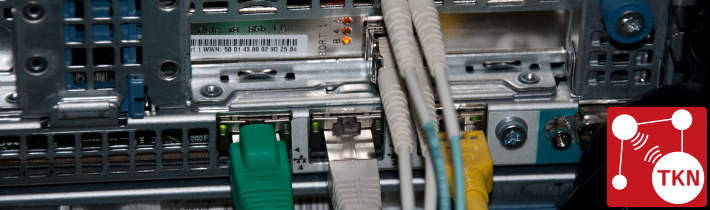
Where to Decide? Centralized vs. Distributed Vehicle Assignment for Platoon Formation
July 17, 2024
Optimized platoon formation is crucial for achieving best performance in terms of travel time and energy consumption. We explore the computation of vehicle-to-platoon assignments based on similarity between vehicles focusing on desired driving speed and current position on the road. We developed three approaches to solve this assignment problem: a centralized solver and a centralized as well as a distributed greedy heuristic. We performed a large-scale simulation study using PlaFoSim to compare all approaches. The centralized solver approach assumes global knowledge and requires a complex MIP solver to compute vehicle-to-platoon assignments. The centralized greedy approach, however, suffers from synchronization and greedy selection effects. Overall, the distributed greedy approach achieves close to optimal results but requires least assumptions and complexity.Julian Heinovski and Falko Dressler, "Where to Decide? Centralized vs. Distributed Vehicle Assignment for Platoon Formation," IEEE Transactions on Intelligent Transportation Systems, 2024. (online first) [DOI, BibTeX, PDF, More details]
Private Over-the-Air Federated Learning at Band-Limited Edge
June 20, 2024
We investigate over-the-air federated learning (OTA-FL) that exploits over-the-air computing (AirComp) to integrate communication and computation seamlessly for FL. Privacy presents a serious obstacle for OTA-FL, as it can be compromised by maliciously manipulating channel state information (CSI). Moreover, the limited band at edge hinders OTA-FL from training large-scale models. It remains open how to enable a multitude of devices with constrained resources and sensitive data to collaboratively train a global model at band-limited edge. To tackle this, we design a novel algorithm PROBE building upon a lightweight over-the-air gradients aggregation rule PB-O-GAR. Specifically, PB-O-GAR combines a random sparsification-like dimension reduction with Gaussian perturbation to provide rigorous privacy and band-adapted communication. It elaborately calibrates the transmission signal according to devices' perceived CSI for heterogeneous power constraints accommodation and CSI attack resilience. We show that by utilizing the common randomness, which deviates from the conventional FL, random sparsification-like dimension reduction can augment privacy in addition to the intrinsic privacy amplification effect of AirComp. We establish near-optimal convergence rates and explicit trade-offs among privacy, communication and utility for PROBE. Finally, extensive experiments on benchmark datasets are conducted to validate our theoretical findings and showcase the superiority of PROBE in realistic settings.Youming Tao, Shuzhen Chen, Congwei Zhang, Di Wang, Dongxiao Yu, Xiuzhen Cheng and Falko Dressler, "Private Over-the-Air Federated Learning at Band-Limited Edge," IEEE Transactions on Mobile Computing, June 2024. (online first) [DOI, BibTeX, PDF, More details]
Leverage Variational Graph Representation For Model Poisoning on Federated Learning
May 15, 2024
This paper puts forth a new training data-untethered model poisoning (MP) attack on federated learning (FL). The new MP attack extends an adversarial variational graph autoencoder (VGAE) to create malicious local models based solely on the benign local models overheard without any access to the training data of FL. Such an advancement leads to the VGAE-MP attack that is not only efficacious but also remains elusive to detection. VGAE-MP attack extracts graph structural correlations among the benign local models and the training data features, adversarially regenerates the graph structure, and generates malicious local models using the adversarial graph structure and benign models' features. Moreover, a new attacking algorithm is presented to train the malicious local models using VGAE and sub-gradient descent, while enabling an optimal selection of the benign local models for training the VGAE. Experiments demonstrate a gradual drop in FL accuracy under the proposed VGAE-MP attack and the ineffectiveness of existing defense mechanisms in detecting the attack, posing a severe threat to FL.Kai Li, Xin Yuan, Jingjing Zheng, Wei Ni, Falko Dressler and Abbas Jamalipour, "Leverage Variational Graph Representation For Model Poisoning on Federated Learning," IEEE Transactions on Neural Networks and Learning Systems, May 2024. (online first) [DOI, BibTeX, PDF, More details]
Low-Complex Synchronization Method for Intra-Body Links in the Terahertz Band
April 16, 2024
The recent literature focuses on deriving analytic channel models for intra-body links through the human tissues, including the analysis of achievable communication capacities in the terahertz band. A yet missing component, however, is a synchronization module to implement communication schemes in the intra-body link. Such synchronization module will ultimately bound the communication performance regarding the perceived signal to noise ratio (SNR) and bit error rate (BER), for instance. This paper contributes to the state of the art in two directions: (a) evaluating the bounds on the communication performance with the Cramer-Rao lower bound (CRLB) for the synchronization symbol timing offset (STO) and (b) designing a low-complex mechanism to synchronize communication.Jorge Torres Gómez, Jennifer Simonjan and Falko Dressler, "Low-Complex Synchronization Method for Intra-Body Links in the Terahertz Band," IEEE Journal on Selected Areas in Communications, vol. 42 (8), pp. 1967–1977, August 2024. [DOI, BibTeX, PDF, More details]
Electric Circuit Representation of the Human Circulatory System to Estimate the Position of Nanosensors in Vessels
March 26, 2024
Nanodevices are the focus of research enhancing the detection and treatment of diseases in the human body. Focusing on the scenario where nanosensors are flowing with the blood in the human circulatory system (HCS), in this work, we investigate a model to predict their distribution along the various vessel segments. Although various approaches report solutions for localizing nanosensors in the body, it is also relevant to derive their stationary distribution along the vessel segments as a prior step to assess their actuation and sensing capabilities in the body. We use a Markov chain formulation to derive the stationary distribution of nanosensors. We evaluate the transition probabilities relying on the representation of vessels with electric circuit components. We implement the electric circuit representation of the left ventricle in the heart and the arteries to find the blood flow at vessel bifurcations and then compute the Markov chain probabilities. Our system also allows to reveal the dynamics of the movement of nanosensors during human activity. We illustrate results in two regimes as low and high activity to mimic the case when being at rest or doing sports.Jorge Torres Gómez, Jorge Luis González Rios and Falko Dressler, "Electric Circuit Representation of the Human Circulatory System to Estimate the Position of Nanosensors in Vessels," Elsevier Nano Communication Networks, vol. 40, pp. 100499, July 2024. [DOI, BibTeX, PDF, More details]
Side Effects of IRS: On the Need for Coordination in 6G Multi-Operator IRS-assisted Networks
February 15, 2024
An IRS manipulates the channel propagation characteristics not only of signals of the operator who controls the IRS, but also of signals of other operators, although they operate at different frequencies. This is because IRS usually do not employ band pass filtering and, thus, also reflect signals of other frequencies. In this paper, we first introduce and discuss the problem of multi-operator coexistence issues that arise in IRS-assisted networks. We then propose splitting a single common IRS into multiple sub-blocks (subIRS) and controlling their dynamic assignment to operators, by taking into account the impact on the proximate operators as well. We show that the performance of the overall multi-operator network can be improved significantly if subIRS are properly assigned to the operators, compared to a purely static or random assignments. Our simulations results reveal that a significant improvement in terms of the sum rate and the fairness with respect to the respective data rate of the operators can be achieved.Joana Angjo, Anatolij Zubow and Falko Dressler, "Side Effects of IRS: On the Need for Coordination in 6G Multi-Operator IRS-assisted Networks," Proceedings of IEEE Global Communications Conference (GLOBECOM 2023), 4th Workshop on Emerging Topics in 6G Communications (6GComm), Kuala Lumpur, Malaysia, December 2023, pp. 1380–1385. [DOI, BibTeX, PDF, More details]
Distributed UWB-based Ranging for Particle Tracking in Avalanches
January 30, 2024
The inner dynamics and transport mechanisms of avalanches - especially on a particle level - remain hidden for most observation approaches. In this paper, we present a particle based, distributed tracking system that is based on ultra-wideband (UWB) ranging and localization. UWB-based positioning is particularly challenging in outdoor scenarios covering large distances in complex topography and fast moving, mobile systems. Our system model considers multiple anchor nodes distributed with inter-node distances in the order of a few hundred meters. Mobile nodes, which move with the avalanche in the field, are tracked via UWB measurements. We present our prototype and demonstrate through first experiments the general feasibility of UWB-based tracking in snow environments.Jonas Kuß, Anselm Köhler, Michael Neuhauser, Rene Neurauter, Johannes Gerstmayr, Jan-Thomas Fischer and Falko Dressler, "Distributed UWB-based Ranging for Particle Tracking in Avalanches," Proceedings of 19th IEEE/IFIP Conference on Wireless On demand Network Systems and Services (WONS 2024), Chamonix, France, January 2024, pp. 125–132. [DOI, BibTeX, PDF, More details]
Empowering the 6G Cellular Architecture with Open RAN
December 01, 2023
In this paper, we highlight the transformative potential of embracing novel cellular architectures by transitioning from conventional systems to the progressive principles of Open RAN. This promises to make 6G networks more agile, cost-effective, energy-efficient, and resilient. It opens up a plethora of novel use cases, ranging from ubiquitous support for autonomous devices to cost-effective expansions in regions previously underserved. The principles of Open RAN encompass: (i) a disaggregated architecture with modular and standardized interfaces; (ii) cloudification, programmability and orchestration; and (iii) AI-enabled data-centric closed-loop control and automation. We first discuss the transformative role Open RAN principles have played in the 5G era. Then, we adopt a system-level approach and describe how these Open RAN principles will support 6G RAN and architecture innovation. We qualitatively discuss potential performance gains that Open RAN principles yield for specific 6G use cases. For each principle, we outline the steps that research, development and standardization communities ought to take to make Open RAN principles central to next-generation cellular network designs.Michele Polese, Mischa Dohler, Falko Dressler, Melike Erol-Kantarci, Rittwik Jana, Raymond Knopp and Tommaso Melodia, "Empowering the 6G Cellular Architecture with Open RAN," IEEE Journal on Selected Areas in Communications, vol. 42 (2), pp. 245–262, February 2024. [DOI, BibTeX, PDF, More details]
Explainability of Neural Networks for Symbol Detection in Molecular Communication Channels
November 05, 2023
Recent research in molecular communication (MC) suggests machine learning (ML) models for symbol detection, avoiding the unfeasibility of end-to-end channel models. However, ML models are applied as black boxes, lacking proof of correctness of the underlying neural networks (NNs) to detect incoming symbols. This paper studies approaches to the explainability of NNs for symbol detection in MC channels. Based on MC channel models and real testbed measurements, we generate synthesized data and train a neural network (NN) model for the detection of binary transmissions in MC channels. Using the local interpretable model-agnostic explanation (LIME) method and the individual conditional expectation (ICE), the findings in this paper demonstrate the analogy between the trained NN and the standard peak and slope detectors.Jorge Torres Gómez, Pit Hofmann, Frank H. P. Fitzek and Falko Dressler, "Explainability of Neural Networks for Symbol Detection in Molecular Communication Channels," IEEE Transactions on Molecular, Biological and Multi-Scale Communications, vol. 9 (3), pp. 323–328, September 2023. [DOI, BibTeX, PDF, More details]
Towards the Simulation of WiFi Fine Time Measurements in NS3 Network Simulator
October 19, 2023
We present FTM-ns3, a software module which implements the 802.11 FTM protocol so that it can be used within the widely used ns3 network simulator. Moreover, we conducted experiments using commodity WiFi-FTM hardware, Intel 8260 and ESP32, and derived empirical error models which can be used in simulations to study the performance of novel FTM-based localization schemes under real channel propagation conditions while taking into account the specifics of the used WiFi hardware and configuration of FTM. Finally, we present results from simulations of a simple localization scheme based on FTM and multilateration which show the great influence of ranging inaccuracy introduced due to multipath propagation in typical indoor environments with line-of-sight (LoS) but strong multipath. Our module is provided to the community as open source and can be easily customized and extended.Anatolij Zubow, Christos Laskos and Falko Dressler, "Towards the Simulation of WiFi Fine Time Measurements in NS3 Network Simulator," Elsevier Computer Communications, vol. 210, pp. 35–44, October 2023. [DOI, BibTeX, More details]
Focusing on Information Context for ITS using a Spatial Age of Information Model
September 26, 2023
In order to cope with the vehicles’ mobility, such information is required to be as fresh as possible for proper operation of cooperative driving applications. The age of information (AoI) has been proposed as a metric for evaluating freshness of information; recently also within the context of intelligent transportation systems (ITS). We investigate mechanisms to reduce the AoI of data transported in form of beacon messages while controlling their emission rate. We aim to balance packet collision probability and beacon frequency using the average peak age of information (PAoI) as a metric. We propose a new way of interpreting the AoI by considering information context, thereby incorporating vehicles’ locations. As an example, we characterize such importance using the orientation and the distance of the involved vehicles.Julian Heinovski, Jorge Torres Gómez and Falko Dressler, "Focusing on Information Context for ITS using a Spatial Age of Information Model," Elsevier Computer Communications, vol. 209, pp. 203–216, September 2023. [DOI, BibTeX, PDF, More details]
TCOA: Triple-Check Offloading Algorithm for Roadside Units and Vehicular Microclouds in 5G Networks and Beyond
August 26, 2023
Next-generation intelligent transportation systems aim to achieve many cooperative perception and cooperative driving functions requiring considerable computational resources. Offloading such tasks via mobile edge computing is considered part of the solution and is currently being investigated in the scope of 5G networks and beyond. In this study, we propose an offloading system architecture to enable such offloading in a vehicular microcloud interconnected by a 5G core network. We model the system as a queueing model to derive closed-form solutions for selected performance metrics. Based on these insights, we propose the triple-check offloading algorithm (TCOA) to obtain both the best offloading ratio to the vehicular microcloud and the optimal maximum of the remaining vehicle instances in the vehicular microcloud. Our simulation results show that the proposed TCOA has better system performance than four other offloading schemes in terms of cost, response time, service rate, and cost response-time production service rate division (CRPSD).Bo-Jun Qiu, Cheng-Ying Hsieh, Jyh-Cheng Chen and Falko Dressler, "TCOA: Triple-Check Offloading Algorithm for Roadside Units and Vehicular Microclouds in 5G Networks and Beyond," IEEE Access, vol. 11, pp. 84985–85001, August 2023. [DOI, BibTeX, PDF, More details]
Load all older featured papers
Last modified: 2023-10-07