Virtual Cycling Environment (VCE)
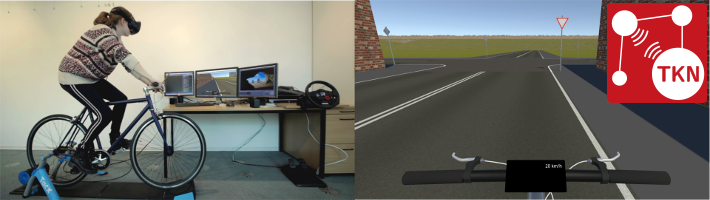

Virtual Cycling Environment for interactive bicycling experiments with vehicle-to-anything (V2X) communication
Summary
In order to trace and record realistic and reliable cyclist behavior, we developed the Virtual Cycling Environment (VCE). It allows cyclists to ride a virtual bicycle in a 3D virtual reality environment by interacting with a physical bicycle on a training stand. Foreign traffic (i.e., cars) and wireless networking are provided by the specialized simulators SUMO and Veins, respectively. The physical bike simulator is then coupled via the Ego-Vehicle Interface (EVI) to this simulation platform. The VCE provides a high degree of realism to the cyclist, thanks to the haptics of a physical bicycle combined with virtual reality systems. Researchers can leverage this to study the interaction of cyclists and their traffic environment without the danger of physical harm. Thanks to the coupling to Veins, even future assistance systems relying on communication can be tested.
If you are using components (or the concept) of the VCE or traces we recorded with the VCE, we would appreciate a citation:Julian Heinovski, Lukas Stratmann, Dominik S. Buse, Florian Klingler, Mario Franke, Marie-Christin H. Oczko, Christoph Sommer, Ingrid Scharlau and Falko Dressler, "Modeling Cycling Behavior to Improve Bicyclists' Safety at Intersections – A Networking Perspective," Proceedings of 20th IEEE International Symposium on a World of Wireless, Mobile and Multimedia Networks (WoWMoM 2019), Washington, D.C., June 2019. [DOI, BibTeX, PDF and Details...]
Sources
- Documentation
- GitHub project
- VCE Bicycle Traces 2019 (zip) [index, md5]
- VCE Bicycle Traces 2019 (tar.bz2) [index, md5]
Press & Media
- Article @ HNI aktuell 01/2019 (14.05.2019)
- Virtual Cycling Enviroment @ YouTube
- Virtual Cycling Enviroment - Intersection Collision Warning via V2X @ YouTube
See also
- Project Virtual Cycling Environment (VCE)
- Project Safety4Bikes